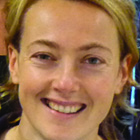
Van der Sluis et al. propose new statistical tool that allows researchers to identify genes associated with multiple phenotypes
TATES: Efficient multivariate genotype-phenotype analysis for genome-wide association studies
Genome-wide association (GWA) studies were designed to search the entire genome for genes that cause individual differences in phenotypes, with the ultimate aim to unravel the biological etiology of physical, medical, and psychological traits. The standard GWA analysis, however, is limited to the study of a single phenotype. Van der Sluis explains why this limitation may be the reason that GWA studies have been less successful in identifying causal genetic variants than was anticipated.
“Researchers generally think that the causes of psychiatric and psychological phenotypes are similar in mechanism to medical diseases. Like the medical disease pneumonia causes symptoms like coughing and fever, depression is believed to be a disease that causes symptoms like worrying, sleeplessness, agitation, loneliness, and sadness. Given this phenotypic model, in which one disorder causes a variety of symptoms, genes are believed to affect the probability to suffer from the general disorder depression. In practice, however, we observe striking differences between patients with the same depression diagnosis: some patients suffer from hyposomnia (sleeplessness), agitated mood, and lack of appetite, while others experience hypersomnia (excessive sleepiness), flat affect, and increased appetite. Such differences in symptomatology are difficult to explain in the standard depression model.‚
“Recently, colleagues at the UvA have therefore challenged this model. Angelique Cramer et al.
for example, argued that depression symptoms might not co-occur because they are all caused by the same psychological illness, but rather because they are mutually causative: worrying causes sleeplessness, lack of sleep causes agitation, agitation causes a bad mood and therefore social isolation, which in turn causes sadness. From this so-called network point-of-view, depression may not be a homogeneous disorder with respect to genetic etiology. Depression may actually be no more than a generic term for a collection of, genetically possibly unrelated, symptoms that often co-occur because they are causally related on a phenotypic level. If this network model is true, then researchers should not look for genes that cause individual differences in the liability to develop “depression‚. In contrast, they should look for genes that cause individual differences in the liability to develop the individual symptoms, while each symptom may be associated with a unique set of genes. Sleeplessness, appetite change, and feeling blue do not necessarily have a single (genetic) cause, as is the assumption under the standard depression model‚.
“Such more complex phenotypic models explain phenomena that the standard depression model cannot explain, like heterogeneity in expressed symptoms. However, the standard genetic methods and software for efficient GWA are not well suited to identify genes for multiple phenotypes (e.g. symptoms) of different genetic architecture. We therefore adjusted a method previously published by Li et al. to allow multivariate GWA analysis. Using extensive simulation, we show that our new method, which we call TATES (Trait-based Association Test that uses Extended Simes procedure) can identify genes that are related to one, some, or all of the phenotypes under study. TATES therefore provides a more comprehensive view of the genetic architecture of complex traits than does the standard univariate GWA analysis. In addition, TATES often has more statistical power to detect genes of small effect than standard multivariate analyses, like MANOVA. We, therefore, believe that TATES provides a viable and powerful new statistical tool for unraveling the biological etiology of complex traits‚.